Website & Mobile App
Project Brief
I led the launch of the Search & Discovery initiative for the Web and App platform. This project involves everything from vendor research, prototyping, designing, and cross-platform coordination to deliver the product.
Responsibilities
UX/UI Design Manager, UX Research Lead, Prototype, Ideation, A/B Testing, Scope Definition
Tools
Figma, FigJam, Miro, JIRA, Algolia
Using customers ' buying and search behaviors, we created personalized search and merchandising placement. 'Recommendations' and 'frequently bought together' performed better than expected on their early testing days and the data gathered from them is helping the team make informed decisions.
Increase in Search
Conversion Rate
Drop in 'No Results'
Search Query
Increase in Click
Through Rate
Decrease in Left Filter
Use for All Categories
Introduction
Search and Discovery - As one of the fastest-growing gourmet food e-commerce businesses, Yummy Bazaar needed to bring its A-game to the search experience. Considering how crucial search and product discovery is, we decided to look for a fast and reliable solution to integrate with.
My Role
My role in the project was to find and implement the most relevant and compatible search product in the market as fast as possible. I was responsible for researching available search products, communicating business requirements to the agency, and communicating technical requirements to my development team alongside leading the design and delivery of the project.
Until early 2023, I was responsible for enhancing the service, adding additional functionalities, and learning from the analytics to deploy improvements.
Customer Insight and
Ideation
Experience Strategy and
Vision
Planning and Scope
Definition
Design Execution and
Validation
I presented research and design work to gain buy-in from stakeholders. I worked on competitive analysis, and wireframes and designed specs to address the problems.
Oversight and Coordination
I designed for responsive web, Android, and iOS devices with a mobile-first approach. I collaborated with my team and the agency's account manager to translate features for each platform.
The Challenge
— SHOW YOU KNOW YOUR CUSTOMERS
The store's search and discovery functionality had big problems. Poor search results meant best sellers were being buried at the bottom of the page while out-of-stock products would appear at the top. With search and filter being weak, customers have to solely rely on navigation. Updating navigation and taxonomy was getting more taxing for the product launch and merchandising team as new products on site were growing exponentially. Often times the search would not be able to show all the results from different datasets, for example, Recipes, Blogs, and Brands page were not shown on search results.
The shopping experience lacked any personalization that customers expect when every other store is competing to make the experience more and more personalized.
In addition, manual merchandising was getting intensive and unscalable for the team. Being remote made the team spend more time on coordination rather than merchandising tasks. With no data on customer search behavior, the team often spends time manually merchandising all the categories periodically without focusing on the bleeding categories.
The main challenges that we are aiming to fix were:
1. Poor search experience and slow load times.
2. Low search relevance leading to poor conversions from search.
3. Unreliable and no analytics for data gathering and merchandising.
Better filter execution with easy-to-use UI and transparency.
The Approach
— RELEVANT, FAST, RELIABLE
I was tasked to find the best available search solution that can integrate with all our platforms and architecture, including the native app and Shopify web structure with easy scalability for future migrations as well.
The assumptions were simple, thousands of customers visit Yummy Bazaar every day. Building a fast and relevant search experience would help customers make faster decisions and reduce friction.
The team had gone through multiple brainstorming sessions and focused on a few key metrics that were perceived to be the most important and advantageous.
Ease of Implementation: We looked for an easy-to-implement solution that can be deployed within a few months and is well-documented.
Support: With working remotely and in different time zones, the requirement for a fast and reliable support team was crucial, as losing sales for a minute could have a big impact on the trust and customer experience.
Innovation: One solution that has multiple products and features that can be added to the primary product, gives us more flexibility to add new features and capabilities faster.
Scalability: Easy-to-understand processes and workflows for the entire team were critical. Multiple teams would be using the new solution for data gathering, merchandising, and other decisions with whole search and navigation. Being able to replicate solutions over product categories and present meaningful data to take action were at the highest stakes.
Relevance: Industry relevance was a very important key proposition that the team discussed thoroughly, during my early research I had gone through numbers on a solution that was providing all the other metrics but their relevance was mostly driven for apparel companies which provided artificially intelligent relevance based on the look and feel of the image i.e. color, shape. patterns, etc. But for the food industry, we had very different categories and search behaviors, addressing those issues early would save us time and resources.
Agile Work Streams
After hours of research and agency calls, we finally pinned down one agency - Algolia. We found a solution for most of the key metrics we were holding onto. The investment looked a bit on a higher scale but the ROI and opportunities on paper were logistically making sense, we just need to execute fast. They had APIs to integrate with several platforms, highly customizable well-documented infrastructure, and other big feature opportunities.
We divided the workflow into two phases and started working on both simultaneously keeping the first phase deliverables first and regularly making progress on the second phase deliverables, we used the Kanban structure to support our schedules.
First-phase deliverables were the ones that can be executed fastest, cookie-cutter features which help with data gathering and migration. The second phase was all the heavy-lifting capabilities that would make us different from others in the industry.
Web Infrastructure
1st
Phase
Auto-complete
Product Listing Page features
Product Detail Page feature
App + Web Infrastructure
2nd
Phase
Auto-correct
Frequently Bought Together
Migrate to app
Personalization
AI Re-Ranking Capabilities
The Discovery
— CUSTOMER INSIGHTS
Research is expensive, for a small startup team with limited resources it could be more of a hassle to research and analyze data in a short period of time. We decided to go with multiple methods which can be tested simultaneously for faster results.
To find customer journey and their pain points, we decided on some mixed research methods to help us better understand the problems and reshuffle our priorities. We conducted interviews with 10 users, and high-scale surveys, and gathered some data with A/B testing.
Triangulation for better UX research
A/B testing: Heatmaps
We conducted some A/B testing on our most search-traffic pages.
User Personas
What do our users look like? And what are their Search and Navigation behaviors?
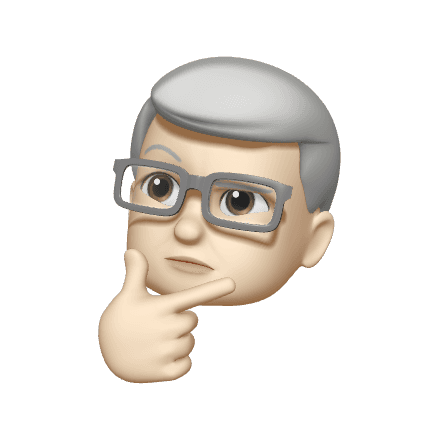
Demographic
A 35-year-old working professional with a busy schedule
Shopping Behavior
Prefers to shop online due to time constraints
Goal
Quickly find specific products and complete the purchase
Search Behavior
Uses the search bar to search for products by keyword, brand or product name
Navigation Behavior
Browses products by categories or filters to quickly find what they are looking for. Prefers to see product photos and descriptions to make informed purchasing decisions.
Frustrations
Gets frustrated when the search results do not provide relevant products or when the product information is incomplete or inaccurate.
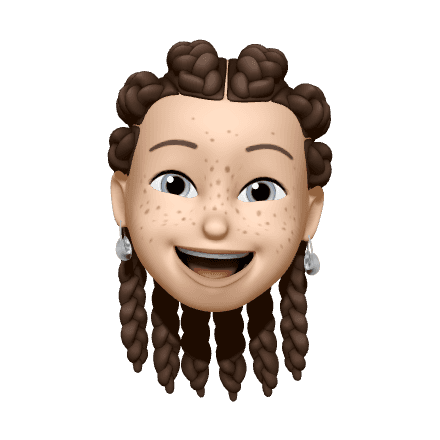
Demographic
A 25-year-old student who enjoys browsing online for products
Shopping Behavior
Enjoys the process of browsing and discovering new products
Goal
Explore a wide range of products and make an informed purchase decision
Search Behavior
Occasionally uses the search bar to look for specific products but mostly relies on category pages and filters to browse products
Navigation Behavior
Prefers to browse products by categories, filters, and subcategories to explore different options. Enjoys reading product descriptions and reviews to gain insights into the product quality and features.
Frustrations
Gets frustrated when the navigation structure is confusing or when the category labels do not accurately reflect the products available. May abandon the site if they do not find the desired range of products or if the product information is not comprehensive enough.
Deeper Insights
With the gathered data, it was clear very early that we had two main user groups who utilizes search and navigation in different ways for their product discovery journey. One customer group was affected more than the other, the existing search was not solving for a busy professional who wanted to search and find relevant products fast.
Poor search results meant best sellers were buried at the bottom of the page, while out-of-stock products appeared at the top.
Low relevance led to poor conversion from search resulting in a higher bounce rate and time spent on the site going through page by page.
With thousands of brands and products, customers were forced to recall the exact item they were looking for in a small attention span.
Products from all over the world also contributed to poor search results based on language nuances and foreign brand products. Resulting in navigation usage and spend more time recalling products
The shopping experience lacked any of the personalization that consumers have come to expect. Some level of personalization with the new search would be easily implemented.
Left Filters for Search and Navigation were very generic and impossible to customize based on the search results resulting in frustrated users.
Reprioritizing our priorities
Web Infrastructure
1st
Phase
Auto-complete
Personalization
AI Re-Ranking Capabilities
App + Web Infrastructure
2nd
Phase
Auto-correct
Frequently Bought Together
Migrate to app
Product Listing Page features
Product Detail Page features
The Vision
Our vision for search and discovery was to create a comprehensive e-commerce platform that combines intelligent search, personalized recommendations, and accessibility to provide a seamless shopping experience for all users.
We should aim to continuously improve the search, discovery, and navigation experience by gathering feedback from users and using data analytics to identify areas for improvement. This includes taking advantage of Algolia's AI capabilities to further add and enhance capabilities.
The Decision
— HOW WE GET THERE
Designing Search & Discovery experiences
Today search and discovery experiences are essential on any e-commerce website, and end-user expectations keep getting higher and higher. At the same time, building experiences with great UI and UX design remains complex and time-consuming. With the help of Algolia's UI Kits, we kickstarted our design faster than ever and started customizing on the go.
Search is more than a search bar
Discovery is everywhere
Detailed Design
For both phases, I went through cycles of requirements, approvals, detailed specs, and handoffs. My process involved sketching and whiteboarding concepts and discussing flows with my team members and then translating them into med-fidelity designs with the help of Algolia's UI kit. UI kits and documents gave us the flexibility to make changes early in the design phase and move forward more quicker.
Autocomplete
Product Listing Page (PLP's)
Detailed Specs
I created two sets of documentation for iOS and Android with different screen sizes during this project to communicate requirements to the development team and support our quality assurance teams in writing test cases.
These deliverables consisted of the technical requirements and the Visual Design Spec later converted on the design systems. This documentation required the most rework during the project and was the highest overhead to maintain.
The Execution
— BRINGING IT ALL TO LIFE
Yb unlocked incremental revenue by customizing the user experience to their specific needs, audience, and catalog. But its first priority was to improve relevance. We tailored the customer experience to their specific needs, audience, and catalog. The team added additional criteria such as product availability, preventing out-of-stock items from showing up first on product listing pages. We used other business data to fine-tune relevance on a query basis, for example improving the average click position on products based on its most keyword 'Italian' which drove high click-through rates. We also took advantage of synonyms to show customers relevant products previously missed. For instance, French-speaking customers searching for 'sirop' get no results, since in the US it's called 'Syrups'. The vendor automatically detected these keywords and we corrected these nuances.
Validation
— TESTING OUR ASSUMPTIONS
After the launch, we doubled down on testing and looking for customer feedback and behaviors. We saw that no results were going down but there are still refinements that were needed to make it better.
We found some obvious issues with keyword ranking and some fine-tuning issues.
Some tags that were allocated before were mistagged and were disturbing to the search results.
Synonyms needed to be allocated to the keywords.
Some fine-tuning included playing with the sort and weight, Auto-complete refinements, gift cards not showing as products, etc.
The Ongoing Refinement
Reducing manual workloads with AI merchandising, while Algolia solutions improved customer experience and improved sales, adding AI merchandising capabilities provided several boosts to the internal teams. The visual merchandising tool came in handy for these merchandising teams. We had a daily touch base and we decided to test a few keywords and see the results improve over time. I had a touch base with my technical team every day to report those issues and test the fixes the next day.
The Launch
— PERSONALIZATION & BEYOND
We launched on the web platform gradually, we wanted to see the customer response and uptime integration with the product. As we see positive responses and data coming through, we did a hard launch 2 days later and launched on the app platform a month later.
With the successful launch, we were looking at some industry-standard metrics and key data points in the hope to improve increased user engagement, higher conversion rates, improved customer satisfaction, better insights into user behavior in the short term, and some competitive advantage in the long run.
The Imapct
Yummy is personalizing the shopping experience to gain incremental revenue and build brand loyalty. The initial implementation was relatively simpler across search and collection pages across the web and app in the second phase.
We created personalized search and merchandising placement using customers' buying and search behaviors. 'Recommendations' and 'frequently bought together' performed better than expected on their early testing days and the data gathered from them is helping the team make informed decisions.
We are also measuring merchandising teams' productivity as the visual editor and other tools provided by Algolia are easy to understand and take action fast.
50%
Increase in Click
Through Rate
Decrease in Left Filter
Use for Category
Reflections
— WHAT I LEARNED
The importance of ongoing optimization and iteration
Launching a new search and discovery engine is just the first step. To truly optimize the user experience, it's important to continually monitor and analyze user behavior, and iterate on your solution based on user feedback and data analytics insights.
The technical complexities of search and discovery
Implementing a search and discovery engine requires a deep understanding of technical concepts such as natural language processing, machine learning, and data analytics combined with an understanding of user behaviors and industry niches. Through this process, I have gained a deeper understanding and the logistics of the technical complexities involved in creating an effective search and discovery engine.
The benefits of cross-functional collaboration:
Implementing a new search and discovery engine required collaboration across multiple teams, including product management, engineering, design, and merchandising. By working together and leveraging each team's unique strengths, we definitely created a more effective and seamless solution.